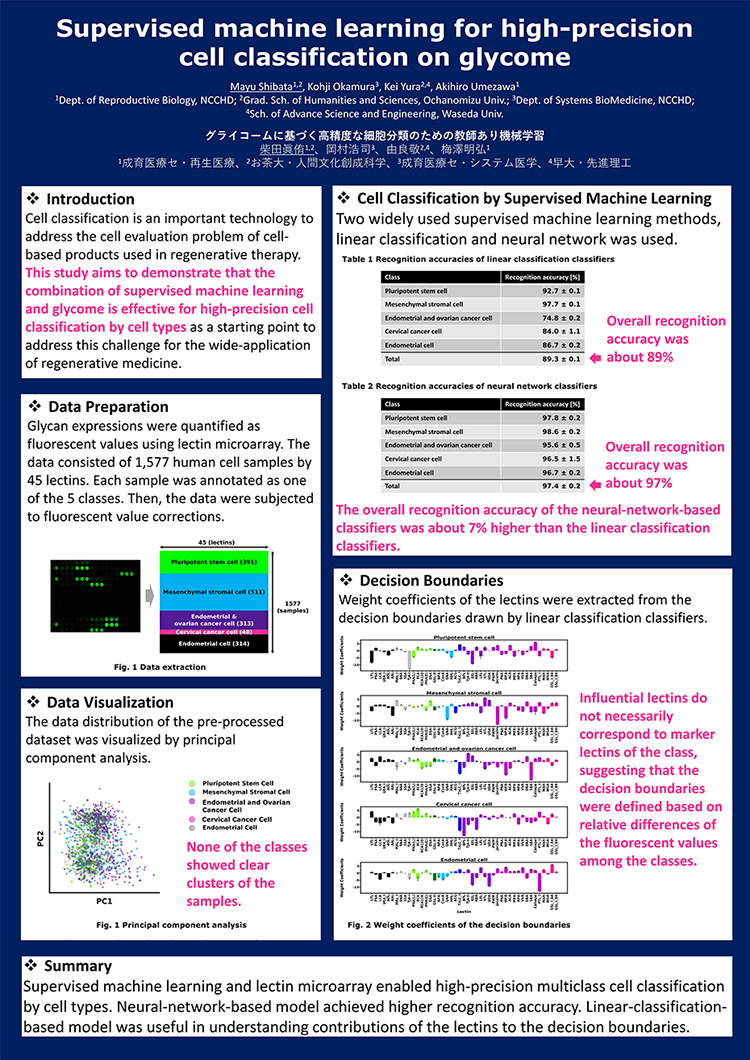
poster_Supervised machine learning for high-precision cell classification on glycome

[PDF:945.5KB]
Tel: +81-3-3416-0181 / Reservation(Hospital): 03-5494-7300
〈Monday~Friday(except national holiday)9A.M.〜5P.M.〉
Cell classification is one of the most important technologies to assure safety and efficacy of the regenerative medicine through evaluation and selection of human pluripotent stem cells (hPSCs) related products. One of the most fundamental questions to establish a platform for quality control of hPSC-based products is to find the method most effective for cell classification. Our previous work showed glycome data, the expression data of the whole glycans expressed in a cell, serve as a rich information source for understanding cells. The objective of this work is to establish supervised machine learning models on glycome data for multiclass cell classification by cell type as a proof-of-concept study. We built supervised machine learning models using two major methods, linear classification and neural network, and tested their performance on the lectin microarray data from 1,577 human cells. The models predicted each sample as a cell from one of the five classes, i.e., pluripotent stem cells, mesenchymal stromal cells, endometrial and ovarian cancer cells, cervical cancer cells and endometrial cells. The linear classification and neural network models achieved recognition accuracies of 89% and 97%, respectively. These high recognition accuracies support our expectation that combination of lectin microarray data and supervised machine learning makes a practical high-precision multiclass cell classification platform. Our work can promote the establishment of quality control system of hPSCs-based products.