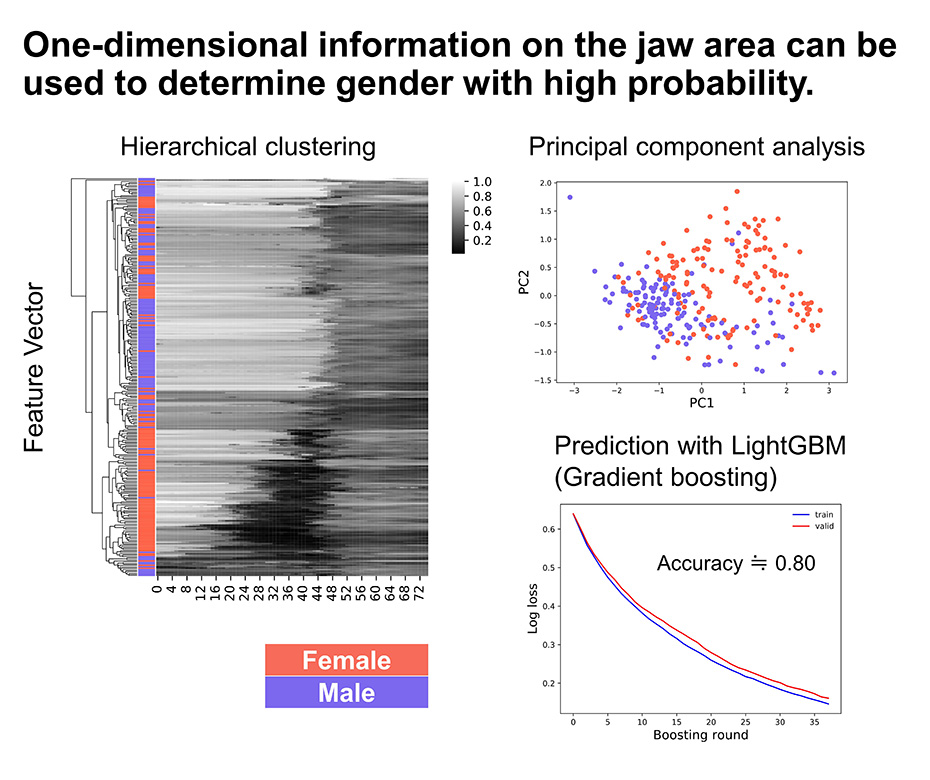
- トップ
- > English
- > Researchers
- > Research Institute
- > Achievement
- > data_science
- > Approaches to Online Collection of Facial Images and Facial Profile Learning by Deep Learning
Approaches to Online Collection of Facial Images and Facial Profile Learning by Deep Learning
Many pediatric genetic diseases that form syndromes are known to have characteristic facial features, and such facial features are important findings that lead to a definitive diagnosis. In fact, a skilled clinical geneticist can identify the name of a disease with a high probability just by taking a glance at the facial expression of a newborn or child. In order to equalize this special skill with experience, various image processing programs have been developed, and in recent years, image recognition combining convolutional operations and deep learning has dramatically improved its accuracy. On the other hand, since deep learning is a machine learning method that relies heavily on the amount of data, it is not easy to collect the desired face images in a specific population. In addition, since many genetic diseases are rare, it is extremely difficult to collect face images to serve as training data. In addition, it is necessary to protect personal information. As a part of the feasibility study of constructing such a diagnostic aid tool, in this study, we collected facial image data from adult volunteers via online and created a judgment model for gender and smile. Since facial images are considered personal information, we constructed an online consent system that does not collect personal information other than the face, i.e., first name, last name, email address, and even gender information, under the approval of the Ethical Review Committee, and collected 2429 facial image data from 277 adult volunteers with and without diseases. The data was collected from 277 adult volunteers with and without diseases. The gender and the degree of smile as the teacher labels for learning were prepared by voting by the members involved in the data analysis. Through cross-validation, it was confirmed that the original model using Inception V3, which underwent data expansion and ImageNet transfer learning, showed recognition accuracy of 96.4% for gender discrimination and 93.0% for smile discrimination. Even when the amount of training data was reduced, and even when the data was limited to images of the jaw area, the recognition accuracy of over 80% was secured. These results will serve as a basis for the development of image data collection methods for rare diseases and diagnostic tools using facial image data.
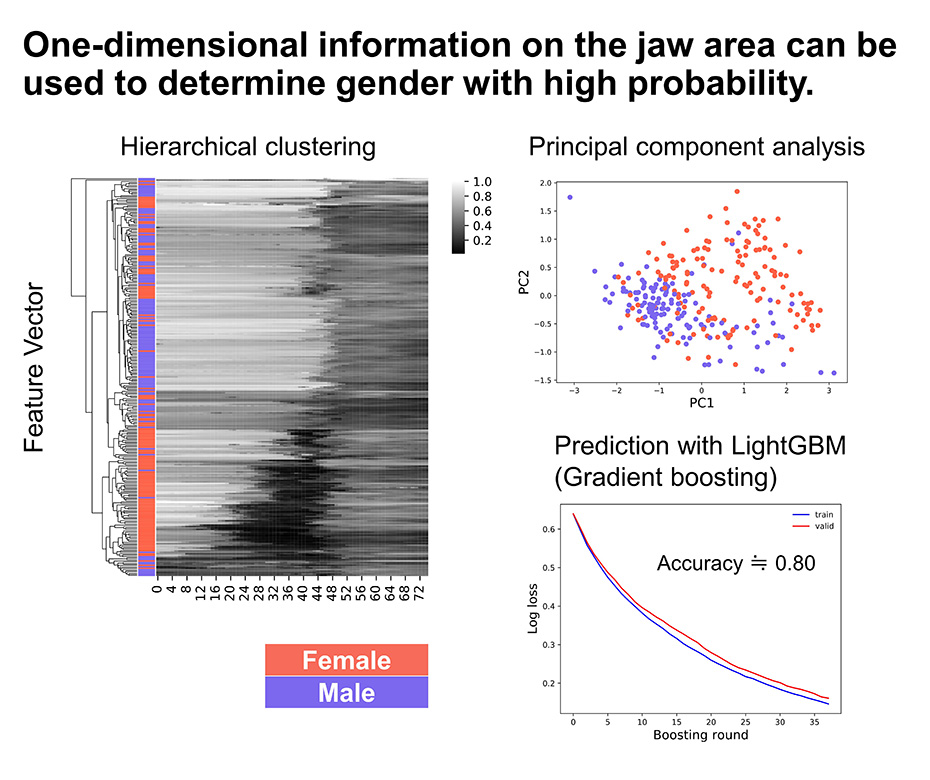